A more perfect union, continued
On to cascaded unions for Shapely ...
>>> from osgeo import ogr >>> from shapely.wkb import loads >>> ds = ogr.Open('/Users/seang/data/census/co99_d00.shp') >>> co99 = ds.GetLayer(0) >>> counties = [] >>> while 1: ... f = co99.GetNextFeature() ... if f is None: break ... g = f.geometry() ... counties.append(loads(g.ExportToWkb())) ... >>> len(counties) 3489
Matplotlib makes a pretty picture of those 3489 polygons:
>>> import pylab >>> from numpy import asarray >>> fig = pylab.figure(1, figsize=(5.5, 3.0), dpi=150, facecolor='#f8f8f8') >>> for co in counties: ... a = asarray(co.exterior) ... pylab.plot(a[:,0], a[:,1], aa=True, color='#666666', lw=0.5) ... >>> pylab.show()
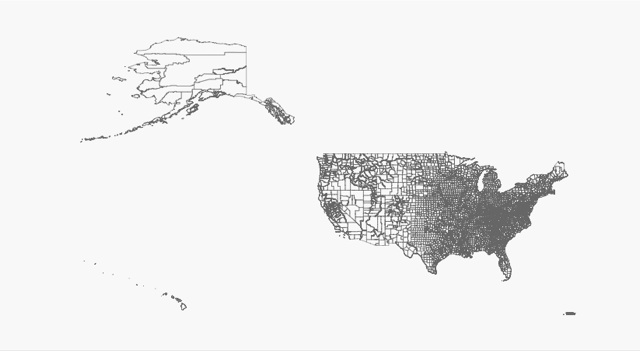
Shapely never had the power to dissolve adjacent polygons in a collection before, or at least not over large collections of real-world data. GEOS 3.1's cascaded unions are a big help:
>>> from shapely.ops import cascaded_union >>> u = cascaded_union(counties) >>> len(u.geoms) 219 >>> for part in u.geoms: ... a = asarray(part.exterior) ... pylab.plot(a[:,0], a[:,1], aa=True, color='#666666', lw=0.5) ... >>> pylab.show()
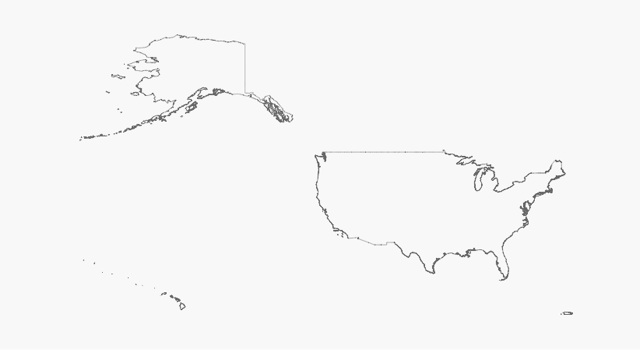
There's user interest in leveraging the new reentrant API in GEOS 3.1, and releasing the GIL when calling GEOS functions to improve performance in multithreaded apps. I'm all for it.